New findings in motion perception
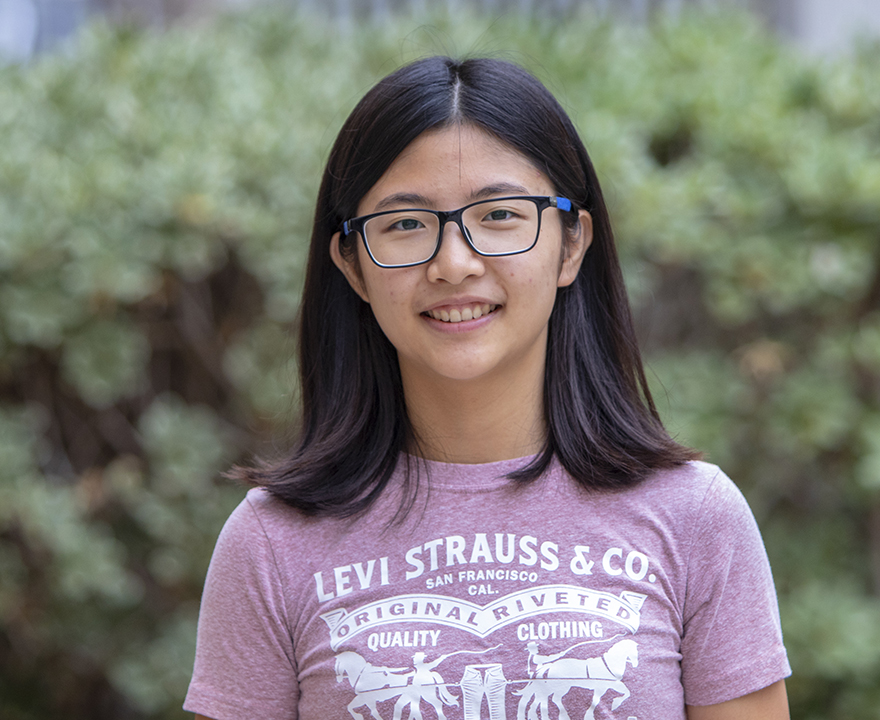
New findings in motion perception
- August 10, 2022
- UCI-led study published in the Journal of Neuroscience
As we move through the environment, our brain must encode our perception of motion rapidly and efficiently. A new UCI-led study published in the Journal of Neuroscience evolves the parameters of a biologically plausible learning rule in spiking neural networks to reconstruct motion patterns. The result is a neural network that can represent complex motion patterns with a reduced number of neurons and less firing activity, says lead author Kexin Chen, UCI cognitive sciences Ph.D. student.
“We developed a computational model whose units showed a remarkable resemblance to real neurons in a motion processing area,” she explains. “This study is important because it suggests how the brain may perceive and efficiently encode motions under biological constraints. Our model, which has a high degree of biological details, provides a test bed for other computational theories of motion perception, and may inspire future studies that seek to bridge between mechanistic theories and biological mechanisms in the brain.”
Coauthors include Michael Beyeler, UCI computer science Ph.D. ‘16 and current assistant professor of psychological and brain sciences at UCSB, and Jeffrey Krichmar, UCI professor of cognitive sciences.
Findings are available online at https://www.jneurosci.org/content/42/30/5882.abstract.
Share on:
Related News Items
- Unlocking consciousness in machines: The groundbreaking journey with UC Irvine's Neurorobotics Lab
- Experts say robots might take your restaurant job - and sooner than you think
- Neurorobotics: Connecting the Brain, Body and Environment
- UCI-led team uses robots to study brain's ability to switch between first-person experience and global map
- Watch a robot cat chase a robot mouse
connect with us