Understanding AI
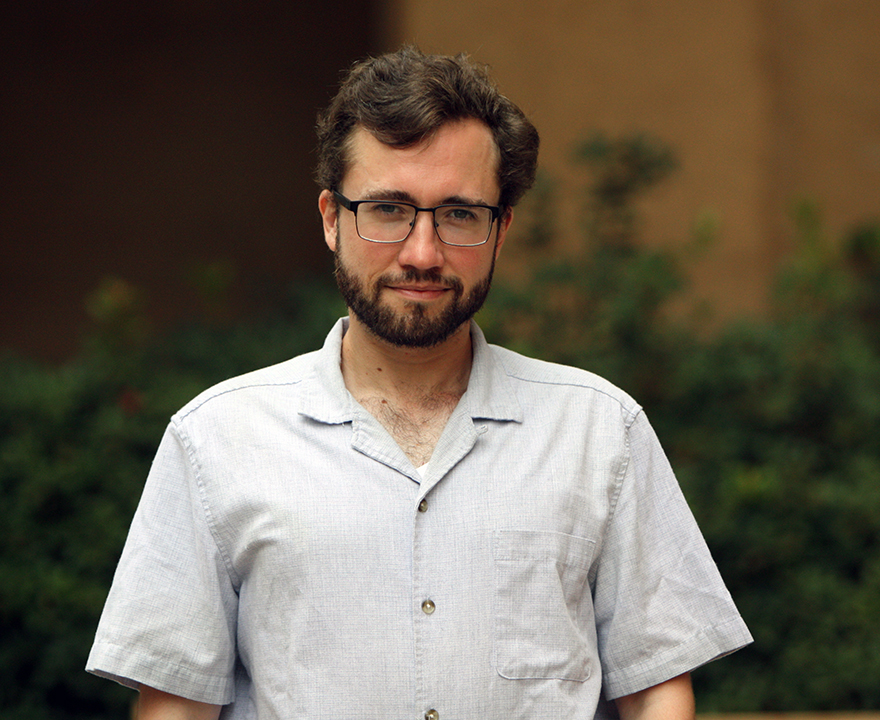
Understanding AI
- August 5, 2020
- UCI language scientist Richard Futrell receives National Science Foundation grant to open the black box of neural networks that guide many of our 21st century devices
Have you ever wondered how Siri and Alexa come to know the answer to many of your most pressing asks? The key, explains UCI language science assistant professor Richard Futrell, isn’t so different from how humans learn: lots and lots of studying, or in the systems’ case, training.
“Natural language processing software – technology we interact with through human language - relies on a machine learning algorithm that looks at a lot of data – text, people speaking – to extract patterns that help it answer questions or have a conversation,” says Futrell. The technology has been around since the 60s but experienced a period of rapid advancement over the last five years as part of the artificial intelligence and machine learning revolution. Now, most approaches rely heavily on neural networks that are trained – not programmed – to deliver responses based purely on patterns extracted from the massive amounts of data they’re fed.
“The current systems are very good,” says Futrell, “and they work better than anything we’ve ever had in terms of performance and accuracy. But they’re only as good as the data that’s used to train their responses, which means there’s an opportunity for things like bias to creep in.”
And because the system learns through training rather than hard coded programming, the computations performed to arrive at an answer aren’t entirely understood by humans.
Taken together, this can be problematic when the machine learning algorithm is applied to processes like determining who gets approval for a mortgage or gendered language used to describe professionals, he says.
With funding from the National Science Foundation’s computer science division, he’s hoping to open the black box of neural networks to determine how they can be understood and controlled. To do so, he’ll rely on methods he’s used in human experiments, similar to tracking eye movement to better understand how text is read and processed by humans.
He’s crafted complex sentences that will be run through the computer driven algorithm which he’ll then monitor as it processes the data to look for patterns of activations inside the network that indicate any surprising sequences.
“Understanding what a ‘surprise’ looks like in the network can help us understand where bias may be present and point to where we might be able to rework the calculation to remove problems,” he says.
Funding will support both graduate and undergraduate research assistants and include training in advanced behavioral and computational linguistics.
“The way we use language is biased, which means training data – collected from real world – is biased,” he says. “As neural networks become an increasingly common way of life in systems that make decisions with large human impact, we need to better understand them and determine how we can remove bias from their learned behavior.”
Funding for this work began in July and runs through June 2022.
-Heather Ashbach, UCI Social Sciences
Share on:
connect with us